华南理工大学计算机科学与工程学院讲座教授、院长华南理工大学科技学院讲座教授他曾任澳门院长(2010-2017) 陈俊龙,受张明明老师邀请,将于2021年3月31日下午14点-15点在会议室中心406会议室开展线下报告,题目为:Dynamic Neural Network Structure: From Incremental Stacked Broad Learning System to Deep Models。
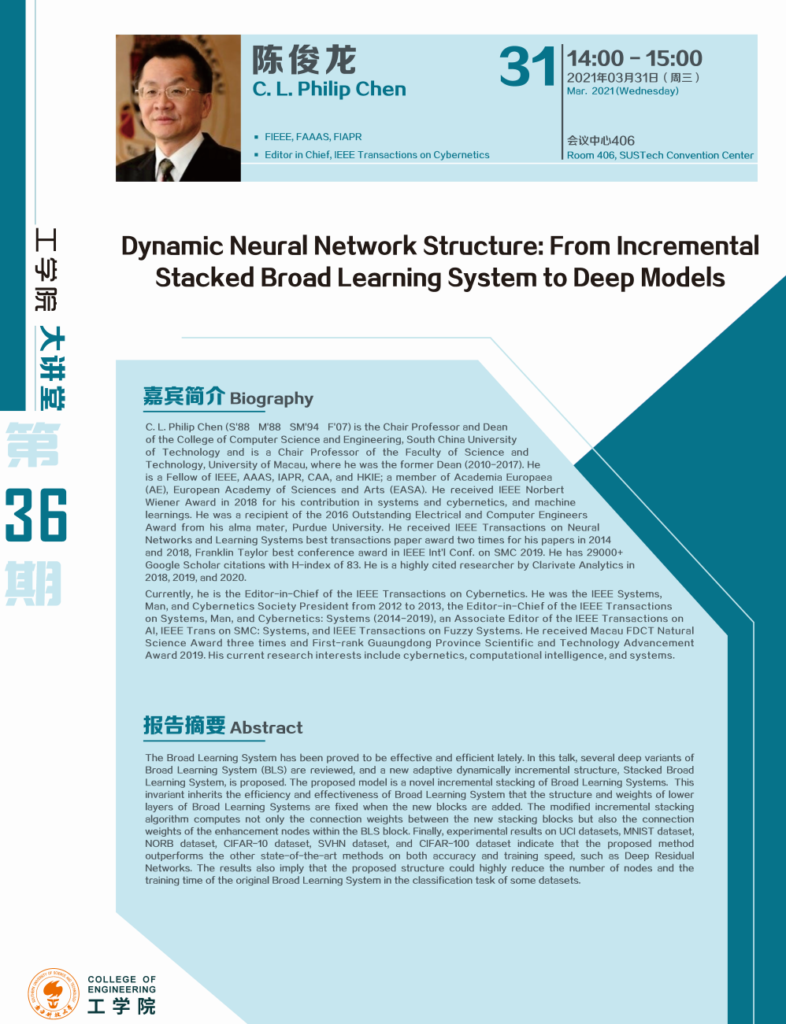
个人简介:
C. L. Philip Chen (S’88 M’88 SM’94 F’07) is the Chair Professor end Deen of the College of Computer Science and Engineering, South China University of Technology and is a Chair Professor of the Faculty of Science andTechnology, University of Macau, where he was the former Dean (2010-2017). He is a Fellow of IEEE, AAAS, IAPR, CAA, and HKIE; a member of Academia Europaea(AE), European Academy of Sciences and Arts (EASA). He received IEEE Norbert Wiener Award in 2018 for his contribution in systems and cybernetics, and machine learnings. He was a recipient of the 2016 Outstanding Electrical and Computer Engineers Award from his alma ater, Purdue University. He received IEEE Transactions on Neural Networks and Learning Systems best transactions paper award two times for his papers in 2014 and 2018, Franklin Taylor best conference award in IEEE Int’l Conf. on SMC 2019. He has 29000+ Google Scholar citations with H-index of 83. He is a highly cited researcher by Clerivate Analytics in 2018,2019,and 2020.
Currently, he is the Editor-in-Chief of the lEEE Transactions on Cybernetics. He was the lEEE Systems,Man, and Cybernetics Society President from 2012 to 2013, the Editor-in-Chief of the EEE Transactionson Systemms, Man, and Cybernetics: Systems (2014-2019), an Associate Editor of the lEEE Transactions onAl, IEEE Trans on SMC: Systems, and lEEE Transactions on Fuzzy Systems. He received Macau FDCT Natural Science Award three times and First-rank Guaungdong Province Scientific and Technology Advancement Award 2019.His current research interests include cybernetics, computational intelligence, and systems.
演讲摘要:
The Broad Learning System has been proved to be effective and efficient lately. In this talk, several deep variants of Broad Learning System (BLS) are reviewed, and a new adaptive dynamically incremental structure, Stacked Broad Learning Systerm, is proposed. The proposed model is a novel incremental stacking of Broad learning Systems. This invariant inherits the efficiency and effectiveness of Broad Learning System that the structure and weights of lower layers of Broad Learning Systems are fixed when the new blocks are added. The modified incremental stecking algorithm computes not only the connection weights between the new stacking blocks but also the connection weights of the enhancement nodes within the BlS block. Finally, experimental results on UC datasets, MNIST dataset NORB dataset, CIFAR-10 dataset, SVHN dataset, and CIFAR-100 dataset indicate that the proposed method outperforms the other state-of-the-art methods on both accuracy and training speed, such as Deep Residual Networks. The results also imply that the proposed structure could highly reduce the number of nodes and the training time of the original Broad Learning System in the classification task of some datasets.